While major white matter tract bundles within the human cortex are largely homogenous in terms of their location and shape, the location of human gray matter areas responsible for coordinating tasks and functions can differ greatly based on the size, shape, and uniformity of an individual's brain.4,5
The core principle of the SCA is that while no two brains are alike, there are common patterns in the way white matter tracts connect parcels together. This means that each parcel should have a predictable “connectivity signature” that can be used to identify it.
The SCA was constructed using three steps: training on healthy brain data, extracting white matter data from patient brain data, and applying the patterns learned on healthy data to the patient data to refine the location of gray matter parcels.
Training requires a large cohort of healthy brain data, without any structural damage or malformation. When applied to healthy brains, the locations and density of white matter tracts, which originate and terminate in gray matter parcels are studied by the SCA to determine the layout of each gray matter region in the atlas.
By first generating a map through Constrained Spherical Deconvolution (CSD), the SCA assesses the location and density of where these tracts originate and terminate - producing a “Structural Connectivity Vector”. The endpoints of white matter tracts are found in small volumes, or “voxels”, of functional gray matter parcels. By assessing these qualities of the white matter, the SCA algorithm generates a pattern for identifying each individual parcel in the atlas, based on the white matter which join them.
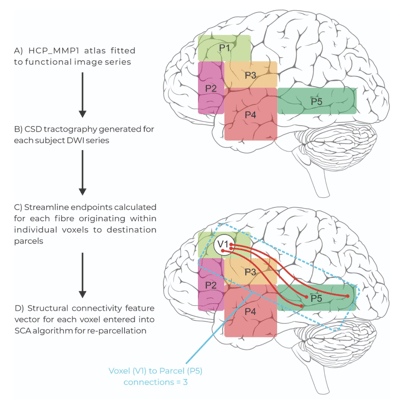
Figure 2: The extracting and application steps of the Structural Connectivity Atlas (SCA) Algorithm. Patient tractography data is entered into the model and voxels containing white matter endpoints are assigned to parcels within the modified human connectome project atlas based on their structural connectivity vector through re-parcellation.
With this pattern identified, the extracting step requires patient brain data. Once again CSD is conducted, and tractography is generated representing the endpoints and density of all white matter tracts within the brain. With patient tractography generated, the SCA asks, “which parcel is this voxel most likely to belong to, given the number of tracts which terminate and originate here?”. An important point of difference to the training step is that rather than collecting information and learning a pattern to identify gray matter, the application step appoints gray matter voxels as their most likely parcel, and in doing so “refines” the atlas from healthy brain data onto patient data in its native space.
Additionally, due to being constrained to a starting set of 379 parcels, the SCA generates reproducible results which can be discussed between patients. In sum, despite the specific locations of gray matter parcels being refined based on individual patient tractography, the end result is a personalized version of the same 379 parcel atlas, enabling valid comparisons for further analysis.